- 비전공자를 위한 HTML/CSS
- Class Aware Sampling
- 이것이 취업을 위한 코딩테스트다
- Semi Supervised Learning
- ComputerVision
- CodeUp6012
- CodeUp6011
- ICLR2021
- Maximum Mean Discrepancy
- 백준
- Vision Transformer
- CVPR2021
- CodeUp6016
- Python
- Consistency Regularization
- Baekjoon
- Domain Adaptation
- ViT
- CodeUp6013
- Computer Vision
- CodeUp6014
- Contrastive Domain Discrepancy
- Feature Augmentation
- 코드업
- CodeUp
- Segmentation Map
- CodeUp6015
- Pseudo Label
- Attention
- transformer
목록Computer Vision (6)
IT 정리용 블로그!
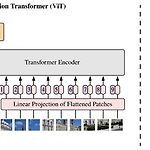
Introduction Self attention 기반의 구조들, 대표적으로 transformer은 NLP에서 좋은 성능을 보인다. Transformer는 연산 효율성이 좋아 매우 큰 크기의 모델들을 학습시킬 수 있었다. 하지만 컴퓨터 비젼 분야에서는, convolutional 구조들이 아직 우세하다. NLP 분야에서의 성적을 보고, CNN 구조를 self attention과 결합하거나 CNN을 완전히 대체하려는 연구들이 진행되고 있다. 본 논문에서는 전형적인 transformer 모델에 거의 수정을 하지 않고 이미지를 직접 적용하는 방법을 실험한다. 이를 위해 이미지를 패치로 나누어 이 패치들의 linear embedding의 sequence를 transformer의 입력으로 사용한다. 이미지 패치들은..
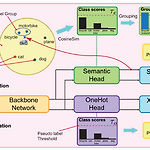
Introduction Semi supervised Learning에 있어 어떤 접근법들은 consistency regularization에 집중하기도 한다. 이들은 동일한 unlabeled input 이미지를 변형한 버젼들에 대해 consistent한 prediction을 내리도록 한다. 반면, 다른 접근법들은 pseudo labeling에 집중하기도 한다. 여기서 모델은 unlabeled data에 대해 인공적인 label을 만들고, 이들을 이용해 train한다. 두 접근법을 섞어 사용하는 것이 SOTA를 보이기도 한다. Pseudo labeling에 있어, 흔히 일어나는 문제는 confirmation bias다. 모델은 잘못된 prediction을 이용해 학습을 진행하고, 여기서 에러가 누적되어 문..
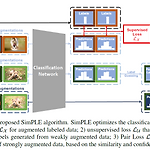
Introduction Semi-Supervised Learning(SSL)의 문제는 제한된 labeled data에서 학습한 정보를 어떻게 일반화하여 unlabeled data로 전할 수 있는가이다. 가까운 데이터는 동일한 label을 share할 확률이 높다는 가정인 continuity assumption을 따라서, Label Propagation을 포함한 많은 접근법이 고안되었다. 서로 다르게 augment된 unlabeled 데이터들 간에 consistency를 만드는 것이 최근 연구동향이다. Consistency regularization을 제외한 몇 다른 방법들도 연구되고 있다. MixMatch, ReMixMatch, FixMatch 등의 방법은 labeled 혹은 unlabeled 데이터에 ..
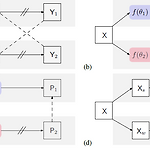
Introduction Semantic segmentation은 픽셀 단위의 training data labeling이 필요하기 때문에 다른 computer vision task보다 비용이 많이 든다. Semi-supervised semantic segmentation에는 Consistency regularization을 많이 사용한다. 이것은 다양한 변화(augmentation 등)에도 예측결과의 일관성을 가져다준다. Self-training도 많이 연구되고 있는데, labeled image에서 train된 segmentation model에서 unlabeled image를 이용해 pseudo segmentation map을 얻고, 이를 training data를 늘리고, segmentation mod..
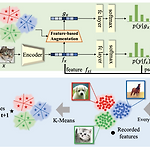
Introduction 기존에 성공적이였던 Semi-Supervised Learning(SSL) 접근법은 image-based augmentation이였다. 하지만 이는 image space에서의 transformation만 가능하고, 다양한 transformation에서의 dataset의 다른 instance의 지식을 leverage해주지는 못한다. 이를 위해 feature-based refinement와 augmentation을 제안한다. dataset에 있는 다른 image feature에서 추출한 prototype의 작은 set을 이용해 image feature에서 soft-attention을 통해 refine과 augment를 하는 모듈을 사용한다. Memory bank와 k-means clus..
Contrastive Domain Discrepancy(CDD) : intra-class discrepancy는 줄이고 inter-class discrepancy는 늘린다. \(P(\phi(X_{s})|Y_{s})\)와 \(Q(\phi(X_{t})|Y_{t})\)의 차이를 측정한다. \(D_{H}(P,Q)\). 평균값의 차를 이용. \(\hat{D}^{c_{1},c_{2}}(\hat{y}_{1}^{t},\hat{y}_{2}^{t},...,\hat{y}_{n_{t}}^{t},\phi) = e_{1}+e_{2}-2e_{3}\). \(c_{1}=c_{2}\)일 때는 intra-class discrepancy 측정. Ø는 feature representation. 첫 feature \(c_{1} \neq c_{..